Destructive Imaging in Particle Beam Microscopy
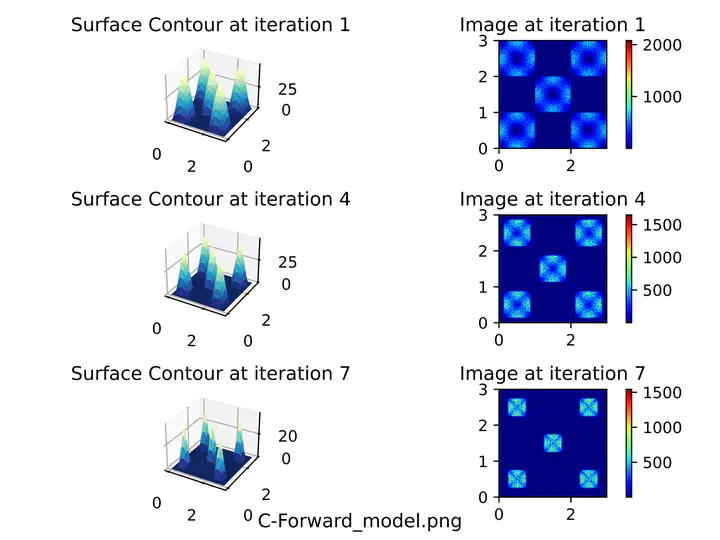
<! In this paper, we discuss a new physics-inspired forward process for imaging samples made from two different materials under a PBM. Under this model, the SE yield is taken to be a measurement of a sample property at that scan location and is distributed as a two-component mixture. We show that this mixture distribution is generally more informative about the SE yield than using a Poisson distribution with a mean given by the beam’s convolution with the sample, as has been done conventionally. We further leverage this mixture model to derive new estimators for edge localization. Our new model allows us to quantitatively give the boundary between the two materials on a grid as opposed to the prior work on post-processing and qualitative improvements on images obtained from the SE counts. We show that the edge position estimation using the Mixture-Model-based estimators can be done more accurately than the beam width used for scanning the sample. We also compare the new edge estimators to an estimator based on the previous convolution-based forward model and show that our new estimators have lesser variance in edge localization on datasets acquired from an SEM. >